Information overload: how investors can make sense of data
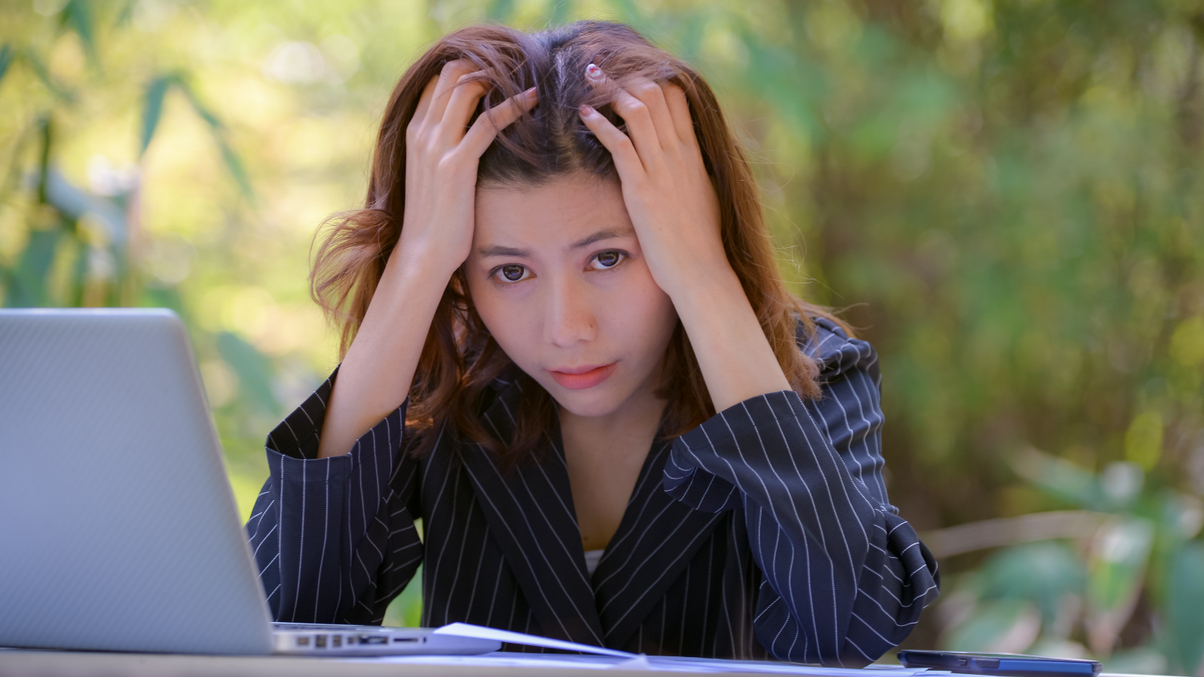
With the rise in popularity of data science, the problem facing fund managers and other institutional investors now is an overload of information rather than a lack of it. Data is increasingly easy to find that the challenge is now making sense of the information gathered, said panellists at AsianInvestor’s COO Investment Week on Tuesday (April 13).
At ‘The devil is in the data’ panel on Tuesday, Muzzaffar bin Othman, chief technology officer at Permodalan Nasional Berhad (PNB) said his biggest challenge is consolidating the data he gets and “having proper definitions".
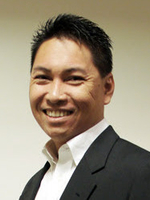
“My problem has always been too much data - the data that we purchase from a third party, the data that we generate from all our analytics, and the data that are generated by departments as well. And these data are then pumped into multiple systems that come up with their own data points, again, after all the processing has been done,” he said.
The issue is compounded by the different stakeholders in the company who have different goals and definitions for the data’s use.
“How do you define each of the data points? Because each department [defines them differently] - the front end will define it according to what they need to see, the middle office will define it based on the operation, and the back office does the same thing,” he said.
“So if you want to look at data from an organisation perspective, we need to have some sort of agreement on what is the meaning of these data points?”
Financial institutions have been moving to integrate data more closely to their investment processes in recent years, with Singapore’s GIC hiring a new head of data science and Zurich Insurance implementing the use of data services to support investment management.
Data analysis has also been shown to improve returns for asset owners and have potential for helping firms meet environmental, social and governance (ESG) goals. However, one area of data use that they were lacking in was artificial intelligence (AI) as asset owners preferred to wait for AI to become more established before investing in it.
To enable cohesiveness in data analysis across departments, bin Othman created a data governance team a year ago that brought together representatives from different departments to discuss and agree on definitions.
“It is, for lack of a better word, a cross functional team who sit down and decide, what is the data that they need? How often do they need it? How do you define those data sets? Where are the sources of errors?” he shared. “Once I understand what those data means to the businesses, then I am able to correlate them, and to do some sort of data warehousing, for all the people to actually have access to it.”
TECH'S INVESTMENT LIMITATIONS
He also eventually also identified three main groups of people and their priorities: management, business, and operations. Management want to see accounting and investment books of record (IBOR and ABOR), while the business group, usually made up of portfolio managers, want portfolio performance numbers, and the operations team need data to work out the settlements and accounting on the backend.
“These three groups need to agree on a similar policy, so to speak, and where the data flows and this data governance team is responsible to put that policy in place and then the execution will be done at the respective businesses,” bin Othman said.
When it comes to using data for investments, he cautioned that “technology is not supposed to do a lot of investment work.”
Instead, “we have own business analysts, technology business analyst to start working with all these three teams, just to make sure and understand what their needs are… We put in quite a lot of good people in technology to understand the business needs to find the right balance,” he said.
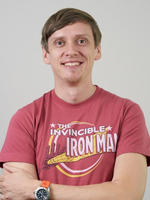
On the other hand, Aki Ranin, co-founder and chief operating officer at fintech company Bambu, which develops robo-advisors, found that he faced difficulty finding the specific data he needed to create predictive models.
“You can’t build AI models to make predictions based on data that you don't have. Maybe the data doesn't exist, maybe the data is in the wrong format. So you'd have to really get creative and think of ways to source, clean, gather, and create these kinds of datasets. That’s definitely a problem that we spend a lot of time and resources thinking about,” Ranin said at the panel.
For example, when they found that the data they needed was not directly available, they had to come up with ideas to get a different type of data from a different source.
He explained that when Bambu was first started up, they wanted to create an AI-driven platform that recommended solutions based on investors’ goals, such as saving for retirement, or to purchase a home.
“It wasn't data that was accessible anywhere, or at least kind of digitally collected,” he said. “So we looked at government data sources on when do people retire, and how many houses are being bought at different prices? And how much are education costs in different countries, and how many people are getting degrees, like bachelor's degrees and master's degrees?”
Based on that data they “generated artificial people with artificial goals” to create models that targeted different age groups and their likely investment goals.
“Generally speaking, when you're doing something new, you're always going to have this problem. If it wasn't done before, then [you have to think harder about] how are you going to start doing it if you're starting from kind of a blank slate,” he said.
AsianInvestor’s COO Investment Week is being held from April 13 to 15, 2021.