Asset owners look to artificial intelligence to fill ESG data gaps
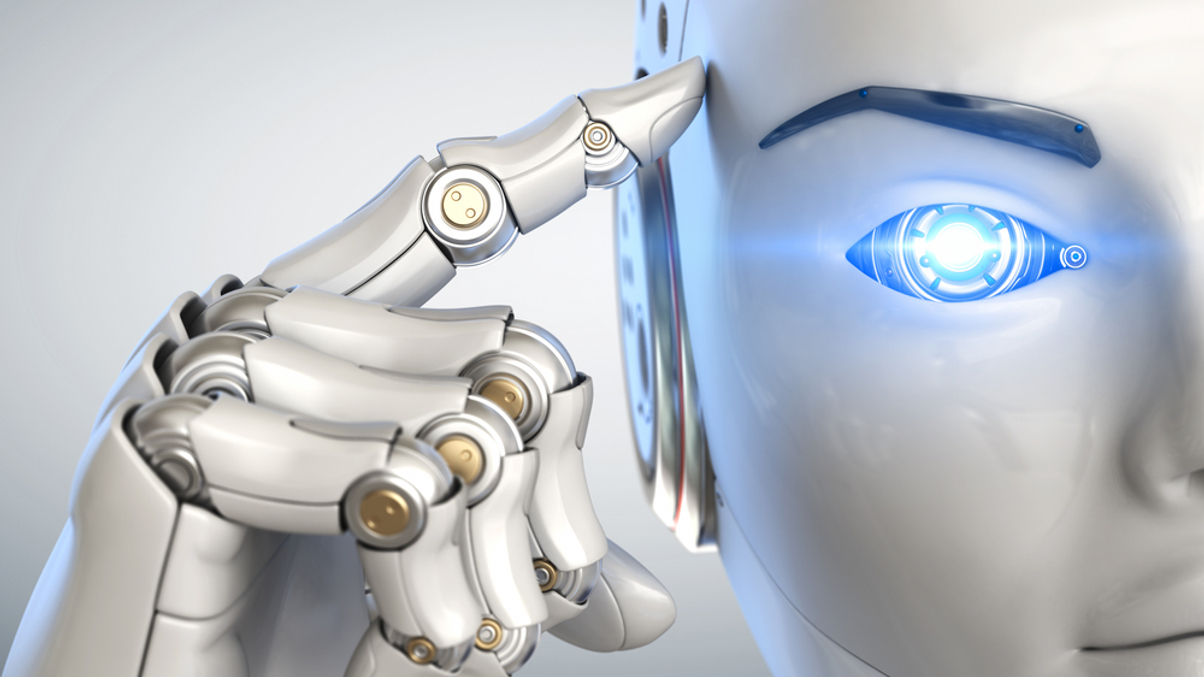
Asset owners are increasingly using artificial intelligence (AI) to collect or infer ESG information across public and private markets, even as they acknowledge the limitations of these new technologies and the importance of human mediation.
AI has played a central role in the development of the ESG taxonomy employed by Sustainable Development Investment Asset Owner Platform (SDI AOP), an initiative founded by AustralianSuper, PGGM, APG and Canada’s British Columbia Investment Management Corporation (BCI) in 2020, whose asset owner members collectively account for $1.5 trillion AUM.
CREATING AN INVESTIBLE UNIVERSE
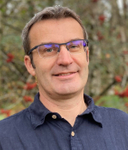
SDI AOP
The AI has helped the taxonomy analyse 9,258 companies to identify those whose products or services contribute to the UN Sustainable Development Goals (SDG), providing asset owner members with an investible universe of equity and fixed income securities that perform well.
Using AI significantly increases the relevant information the taxonomy can be applied to. Last year, for example, the AOP started to measure companies’ patent portfolios against the SDGs.
“The AI scans records of 20 million patents that are updated every week, a task that would be impossible for a human team,” said James Leaton, head of research at SDI AOP.
The AI scans accounts and company publications, as well as other publicly available information, for constituents of a range of equity and fixed income indices – work that would require a huge team of human staff.
“The AI also allows us to collect more granular information than basic sector classification,” Leaton said. Revenue streams of a power company, for example, may not distinguish coal revenues from renewables revenues; the AI can search other company publications to fill the gap, he noted.
Leaton said that human researchers point the AI in the direction of pertinent information, noting where in an SEC filing to find information about products for a US company, for example.
INFERRING POWER USE
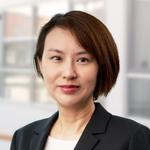
Allianz RE
Suat Ghee Ong, head of asset management in Asia at Allianz Real Estate in Singapore, who manages its decarbonisation project in Asia, told AsianInvestor that the company was exploring AI to help collect data from its residential buildings in Japan.
Energy usage data from Japanese residents is notoriously hard to collect since each tenant is in charge of their own energy procurement.
“We are considering AI technology to measure tenant emissions so that we have visibility over the breakdown of usage while respecting their data privacy and staying within local regulations,” she said.
Tomomi Shimada, lead APAC sustainable investing strategist at J.P. Morgan Asset Management, said the company had developed language processing tools as part of a proprietary machine learning engine that helps its team discover and distil a wide range of material ESG information, drawing on complex data and a range of information sources.
EVALUATING SOCIAL IMPACT
“The data gaps exist across the whole ESG spectrum but our tools are mostly used in the social sphere. We work on a number of social factors where it is difficult to obtain quantifiable information,” Shimada said.
“Some Chinese companies, particularly smaller firms that are new to corporate sustainability reporting, may only release ESG information in Chinese and/or on a selected set of social media, which may be easily missed by third-party global data vendors and ESG ratings providers,” she said, adding that the company worked with China-specific data vendors to expand their coverage of disclosures and alternative datasets, including Chinese-language social media.
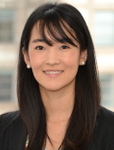
JP Morgan AM
LIMITATIONS OF AI
All those that AsianInvestor contacted stressed the limitations of AI, the importance of human oversight to maximise its efficacy and prevent it from making mistakes, and the superiority of actual, over inferred, information.
“For example, you want to ensure the AI doesn’t confuse strong disclosure for strong performance against the SDGs,” said SDI AOP’s Leaton.
“If you are looking more at sustainability disclosures, that means ensuring companies that don’t disclose information about activities contributing to SDGs (often the case with smaller companies in Asia, for example) are not penalised for this relative to those that do - a large European company, for example.
Despite the potential provided by AI, the best way to fill the gaps in a building's ESG footprint was with real data, analysts said.
“To be sure, machine learning is not a substitute for actual company disclosures,” said Shimada, stressing the need to combine information collected by AIs with human insight.